Researchers from the University of Texas at Austin have developed a noninvasive AI system that can translate a person’s brain activity into a stream of text. The peer-reviewed study was published in the online journal Nature Neuroscience on Monday, May 1.
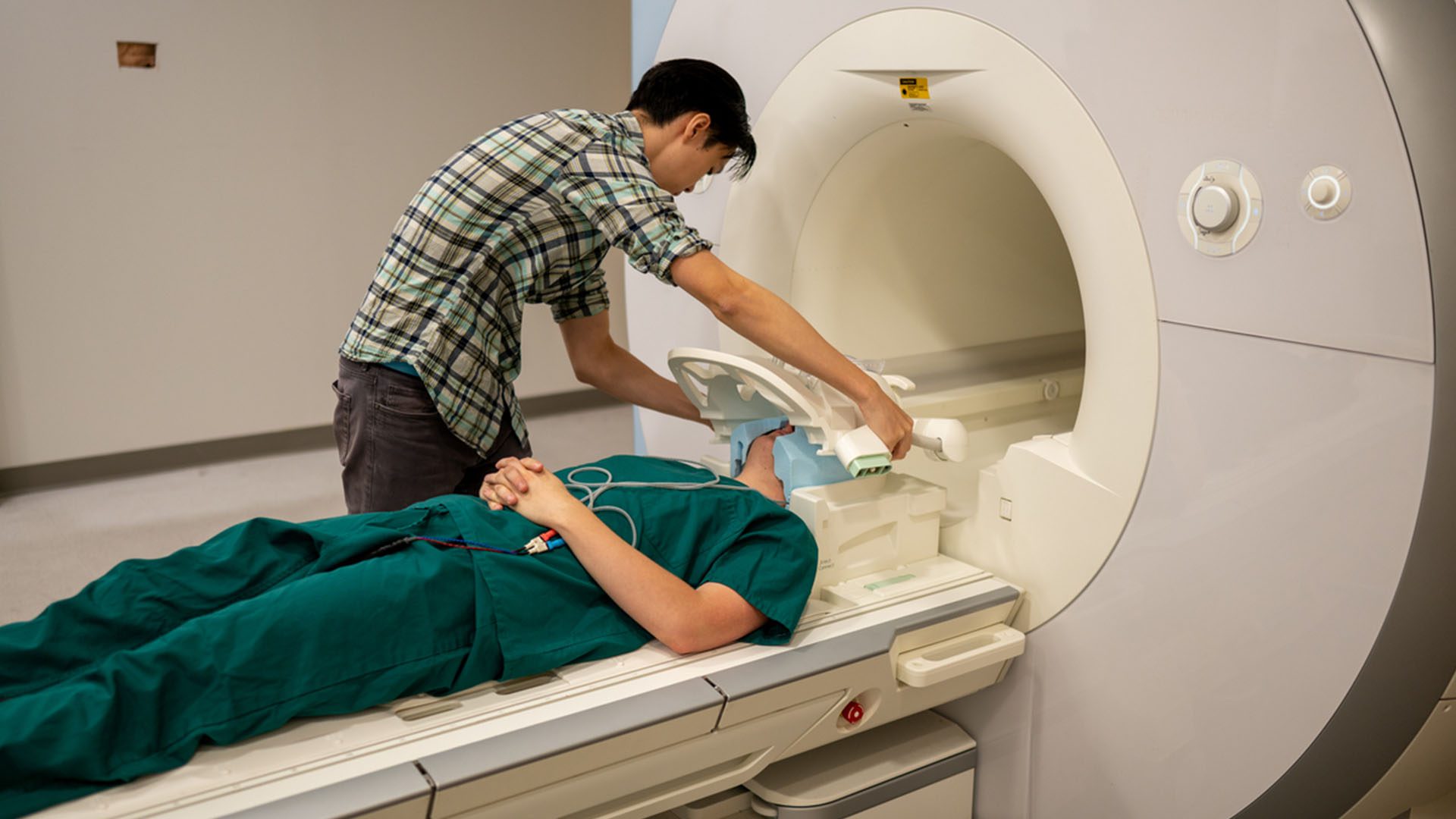
About the AI System
The noninvasive AI system is called a semantic decoder. To train the decoder, the study’s three participants listened to sixteen hours of podcasts each while in an fMRI scanner. The decoder was then trained to match the participants’ brain activity to meaning by using the large language model, GPT-1. GPT was fine-tuned by using over 200 million total words of Reddit comments and 240 autobiographical stories from the podcasts The Moth Radio Hour and Modern Love that were not used for decoder training or testing.
Once the system was trained, the same participants were scanned listening to a new story or imagining telling a story; the decoder could generate corresponding text from brain activity alone. About half of the time, the trained decoder system produced text that closely matched the intended meanings of the original words. The result was not a word-for-word transcript; instead, the text captured the intent of the general thoughts of ideas. For example, when a study participant heard, “I don’t have my driver’s license yet” during an experiment, the thoughts were translated to, “She has not even started to learn to drive yet.”
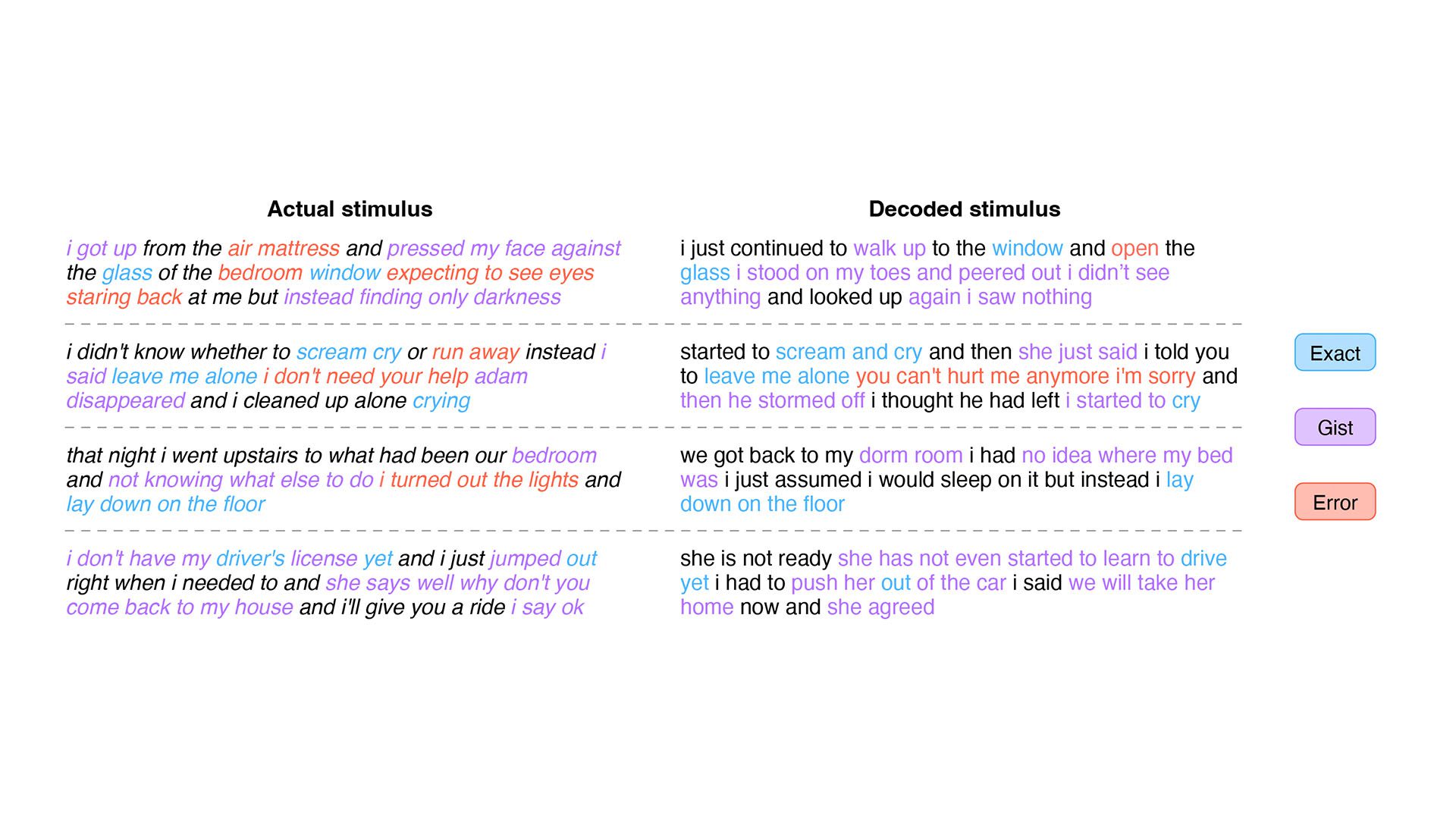
Unlike previous language decoding systems, this study did not require surgical implants and involved corresponding a string of words with a particular meaning. As Alexander Huth, one of the leaders of the study, explained, “For a noninvasive method, this is a real leap forward compared to what’s been done before, which is typically single words or short sentences. We’re getting the model to decode continuous language for extended periods of time with complicated ideas.” The study’s leading researchers have filed a Patent Cooperation Treaty patent application for the technology.
Participants also watched four videos without audio while in the scanner. Similarly to the rest of the study, the semantic decoder was able to accurately describe certain events from the video by just using the participants’ brain waves.
Study Applications & Concerns
The system is currently only operable inside the laboratory because of its reliance on the time needed on an fMRI machine. However, researchers believe that this system could work on other portable brain-imaging systems, such as functional near-infrared spectroscopy (fNIRS). Just like fMRI, fNIRS measures where there’s more or less blood flow in the brain at different points in time.
Especially if the system becomes portable, it could eventually help patients who are mentally conscious but have lost their ability to physically speak, such as those suffering from a stroke or paralysis.
The peer-reviewed study and corresponding press release also addressed some of the concerns that may arise with this type of AI-powered device. For example, this technology can not be used on someone without them knowing—the system must be extensively trained for up to 15 hours while a person is lying in an MRI scanner, being perfectly still, and paying close attention to the stories they are listening to. Additionally, people can actively resist the system’s attempts at decoding their brains. The researchers found that if participants thought of animals or imagined their own stories, the system could not recover the speech the person was exposed to.